Doctoral Defense by Olivier Pauly
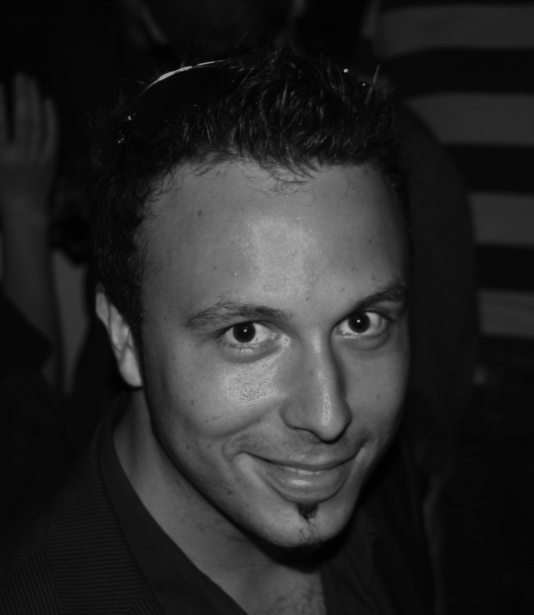
|
- Date: Thursday August 30th, 2012
- Time: 10:30
- Location: Room 00.05.035, FMI-Building, Garching, Boltzmannstr. 3
|
Random Forest for Medical Applications
Abstract:
Machine learning incarnates a key component for integrating the knowldege and experience of physicians into medical imaging applications. Random forests are a popular learning algorithm consisting in an ensemble of independent decision trees. They are very intuitive models that offer a flexible probabilistic framework for solving different learning tasks. In this thesis, we formalize random forests models as ensemble partitioning approaches and propose novel related techniques for classification, regression and clustering. We introduce new task-specific forest models and demonstrate their great potential in different medical applications such as organ localization, segmentation and image categorization.