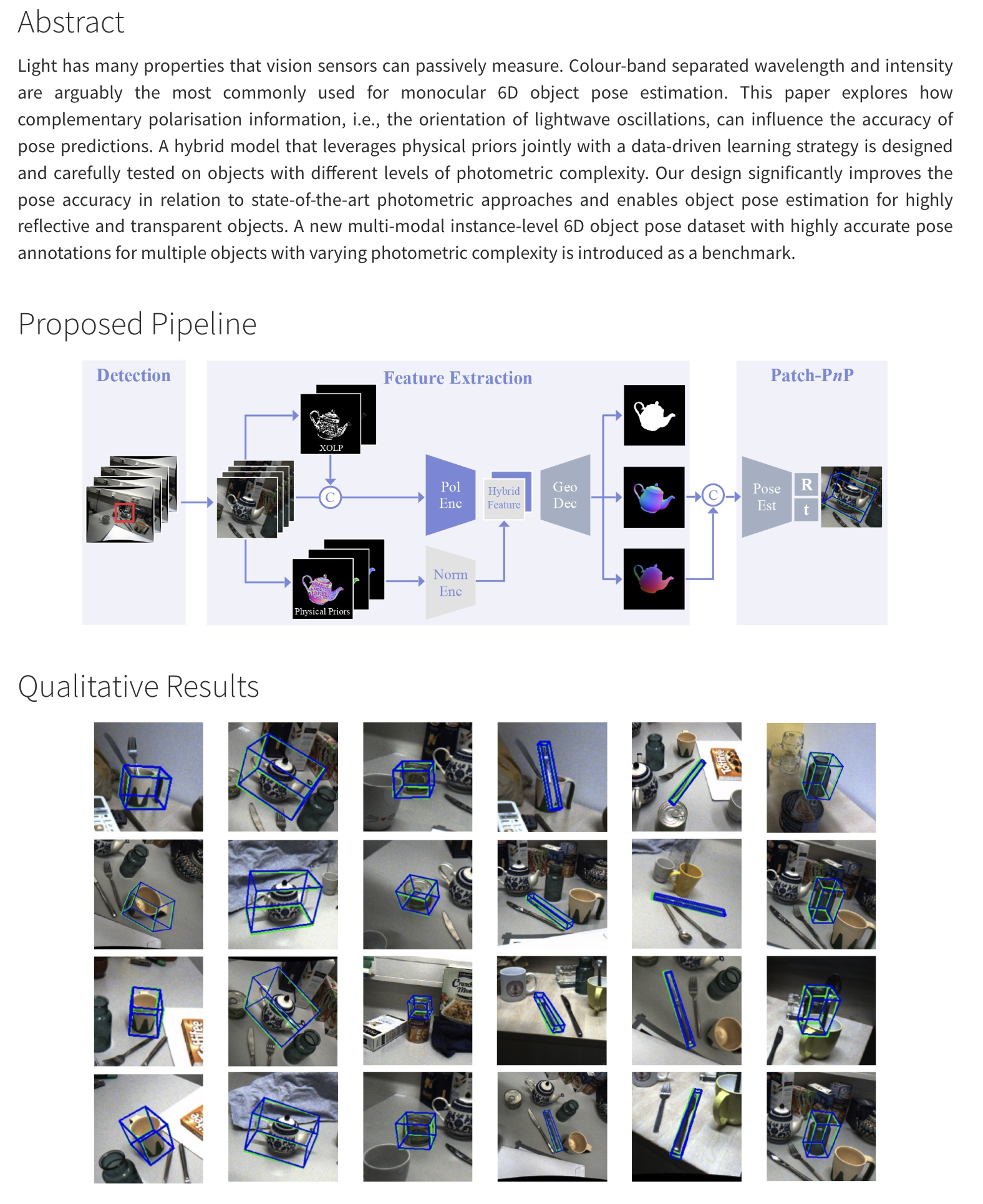
Introduction
Light has many properties that vision sensors can passively measure. Colour-band separated wavelength and intensity are arguably the most commonly used for monocular 6D object pose estimation. This paper explores how complementary polarisation information, i.e., the orientation of lightwave oscillations, can influence the accuracy of pose predictions. A hybrid model that leverages physical priors jointly with a data-driven learning strategy is designed and carefully tested on objects with different levels of photometric complexity. Our design significantly improves the pose accuracy in relation to state-of-the-art photometric approaches and enables object pose estimation for highly reflective and transparent objects. A new multi-modal instance-level 6D object pose dataset with highly accurate pose annotations for multiple objects with varying photometric complexity is introduced as a benchmark.
Download
The dataset is published under CC-BY-NC license and is free to use for non-commercial purposes. Link
Citation
If you use the dataset for research, please consider citing:
@InProceedings{gao2022ppp,
title = {Polarimetric Pose Prediction},
author = {Daoyi Gao and Yitong Li and Patrick Ruhkamp and Iuliia Skobleva and Magdalena Wysocki and HyunJun Jung and Pengyuan Wang and Arturo Guridi and Benjamin Busam},
booktitle = {European Conference on Computer Vision (ECCV)},
year = {2022},
month = {October}
}