Publications
2020 |
|
M. Slavcheva, M. Baust, S. Ilic
Variational Level Set Evolution for Non-rigid 3D Reconstruction from a Single Depth Camera
IEEE Transactions on Pattern Analysis and Machine Intelligence (PAMI). The final publication is available at ieeexplore.ieee.org. (supplementary)
(bib)
|
2018 |
|
C. Kozlov, M. Slavcheva, S. Ilic
Patch-based Non-rigid 3D Reconstruction from a Single Depth Stream
International Conference on 3D Vision (3DV), Verona, Italy, September 2018
(bib)
|
|
M. Slavcheva, M. Baust, S. Ilic
SobolevFusion: 3D Reconstruction of Scenes Undergoing Free Non-rigid Motion
IEEE/CVF Conference on Computer Vision and Pattern Recognition (CVPR), Salt Lake City, USA, June 2018 [spotlight] (supplementary)
(bib)
|
2017 |
|
M. Slavcheva, W. Kehl, N. Navab, S. Ilic
SDF-2-SDF Registration for Real-time 3D Reconstruction from RGB-D Data
International Journal of Computer Vision (IJCV), Springer Verlag. The final publication is available at www.springerlink.com.
(bib)
|
|
M. Slavcheva, M. Baust, S. Ilic
Towards Implicit Correspondence in Signed Distance Field Evolution
PeopleCap Workshop, IEEE International Conference on Computer Vision (ICCVW), Venice, Italy, October 2017
(bib)
|
|
M. Slavcheva, M. Baust, D. Cremers, S. Ilic
KillingFusion: Non-rigid 3D Reconstruction without Correspondences
IEEE Conference on Computer Vision and Pattern Recognition (CVPR), Honolulu, USA, July 2017 [spotlight] (supplementary)
(bib)
|
2016 |
|
M. Slavcheva, S. Ilic
SDF-TAR: Parallel Tracking and Refinement in RGB-D Data using Volumetric Registration
British Machine Vision Conference (BMVC), York, UK, September 2016 (abstract)
(bib)
|
|
M. Slavcheva, W. Kehl, N. Navab, S. Ilic
SDF-2-SDF: Highly Accurate 3D Object Reconstruction
European Conference on Computer Vision (ECCV), Amsterdam, The Netherlands, October 2016 (supplementary)
(bib)
|
Teaching Assistance
|
|
Datasets
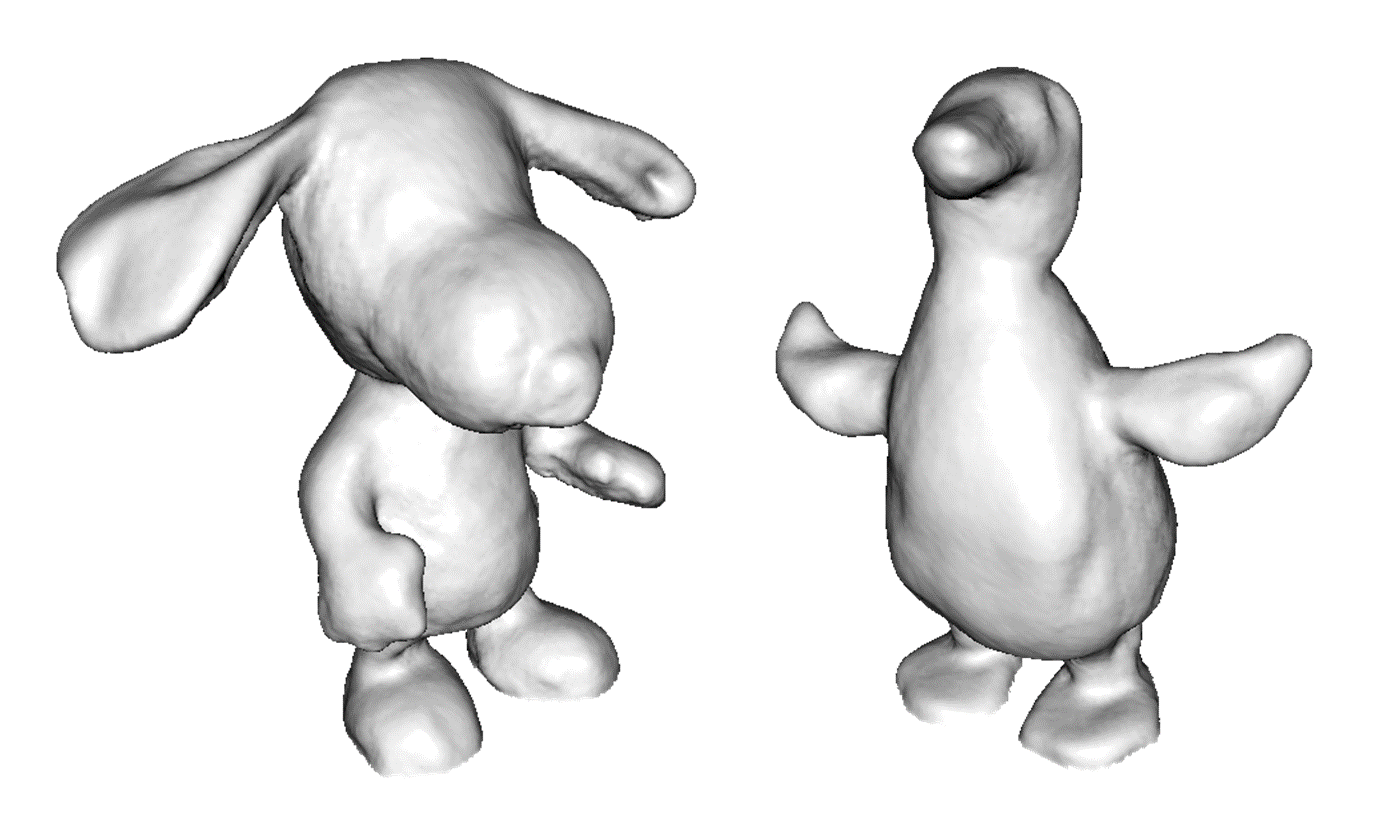 |
|
The single-stream RGB-D sequences with ground-truth canonical pose 3D models we acquired for our KillingFusion? paper are available here.
|
The 3D-Printed Dataset introduced in the SDF-2-SDF paper is available here.
It contains groundtruth CAD models and camera trajectories for 5 objects, each of which is scanned both with turntable and handheld motion. We provide synthetic, industrial- and Kinect-quality RGB-D sequences.
|
|
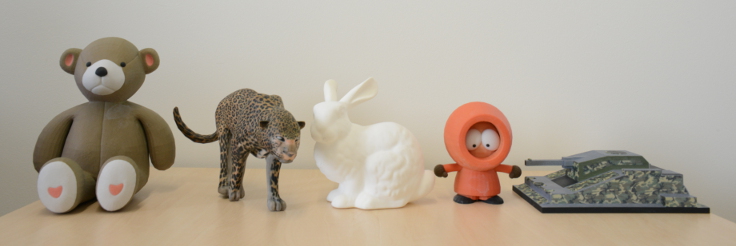 |
Projects
Implicit-to-Implicit Registration for Rigid-Body Motion Estimation
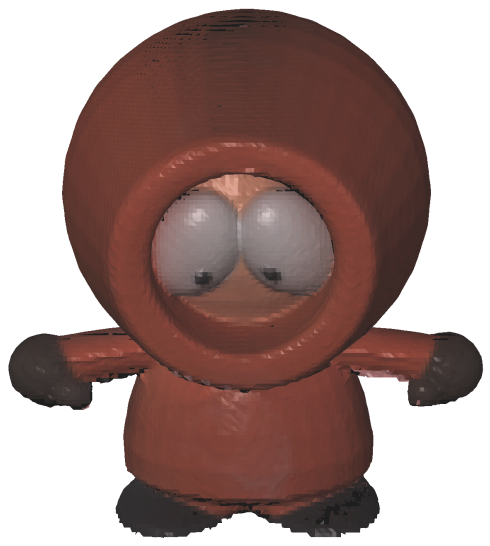 |
|
This is a method for precise registration from range data, in which pairs of signed distance fields are aligned by means of minimization of their direct per-voxel difference. Some of the advantages over cloud registration techniques are the absence of correspondence search and the denser formulation, which lead to higher accuracy and a larger convergence basin.
It is used as the basis for SDF-2-SDF, an object reconstruction pipeline that combines frame-to-frame camera tracking and multiview pose optimization, and SDF-TAR, an extension to larger scenes and SLAM.
The project started with my master thesis (Unified Pipeline for 3D Reconstruction from RGB-D Images using Coloured Truncated Signed Distance Fields), supervised by Wadim Kehl. It was selected for presentation at the Young Researcher Forum at GCPR 2015. Later, I got a chance to present the underlying approach as an oral at the WiCV workshop at CVPR 2016 (poster).
|
Signed Distance Field Evolution for Non-rigid 3D Reconstruction
This is a variational framework for 3D reconstruction of non-rigidly moving surfaces captured with a single RGB-D camera. We estimate a dense deformation field that warps a given SDF to a target SDF, steered by a data term that enforces voxel-wise alignment. To ensure plausible shapes and impose regularity over the field, we propose different strategies, such as the approximately Killing vector field regularizer used in KillingFusion, and the gradient flow in Sobolev space used in SobolevFusion. The advantage of these SDF-based evolution schemes is their ability to handle topological changes and rapid motions, which are challenging for mesh-based techniques. However, this entails loss of data association.
To recover correspondences, we study different possibilities with the lowest-frequency eigenfunctions of the Laplacian matrix representing the SDFs. For moderate motions, we are able to estimate data association implicity via a Laplacian eigencolour term in the evolution energy. For larger motions it is not sufficient, so we explicitly determine correspondences via signature matching of lower-dimensional embeddings of the eigenfunctions. Although the estimated correspondences are not always as reliable as those yielded by approaches that rely on correspondences for the deformation field calculation, we believe that this is a neat way to incorporate data association in a framework that is able to handle changing topology.
|
|
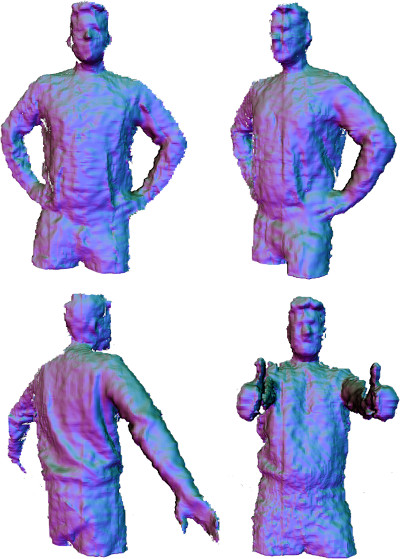 |
|